Neuroinformatics
- Investigate how neural information can be controlled through AI
- Elucidate the information representation of neural activity using big data containing human intracranial electroencephalogram (EEG) signals with AI
- Develop a technology to decode neural information for clinical application as Brain-Machine Interface (BMI)
- Combine the technology to decode neural information with neurofeedback to induce neural plasticity for clinical application
- Elucidate the motor control mechanism using a dynamical system
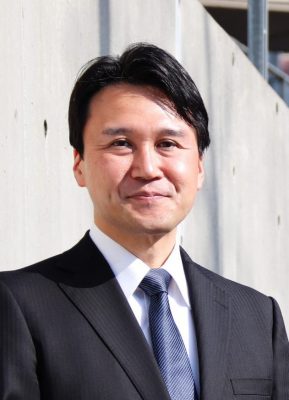
Elucidating the neural information representation to develop a technology to decode and control neural information for medical application
1. Brain-Machine Interface
Neural information related to human intention, visual cognition, and conception can be elucidated by applying machine learning (artificial intelligence) to big data of physiological signals such as EEG (Nat. Comm. 2024). We have developed a technology to decode neural information using intracranial EEG signals measured with electrodes placed in the human brain, and have leveraged this technology to develop a brain-machine interface (BMI) that controls robots and presents self-generated images on screen (Ann. Neurol., 2012, Comm. Biol., 2022, Figures A, B). This technology can be used for medical application, helping immobile patients restore motor function and express intentions.
2. Neurofeedback
BMI can be used to connect robots and avatars in place of human bodies with neural activity to induce plastic changes and reveal the relationship between neural activity and function related to motor control. We have also demonstrated that BMI-driven robot training can reduce phantom limb pain, in which patients feel pain in their missing arm after amputation (Nat. Comm. 2016, Neurology 2020, Figure C). This is called neurofeedback (NF) therapy. By decoding neural information, we will develop NF therapy using the information.
3. Decoding neural information from EEG signals
The technology to decode EEG signals can be applied to diagnose dementia and epilepsy (Neural Net. 2024). The macrodynamics of neural activity can be derived through mathematical elucidation of neural information representation in EEG signals (Comm. Biol. 2023). We will elucidate neural information representation using AI and new mathematical methods.
4. Neuroinformatics
We will contribute to maintain and improve human health using biological signals routinely measured with wearable sensors and other tools. Neural information contains a wide variety of physiological signals to help predict human conditions, including not only EEG but also electromyogram (EMG) and motion analysis data. We aim to utilize neural information for diverse medical application, from health improvement to neural function restoration, by developing big data of extensive biological signals ranging from invasive intracranial EEG signals to those measured with wearable sensors as neural information and decoding and controlling the information. To this end, we are striving to develop human resource that can conduct not only medical research but also interdisciplinary research spanning different fields such as informatics, engineering, and neuroscienceatical elucidation of neural information representation in EEG signals (Comm. Biol. 2023). We will elucidate neural information representation using AI and new mathematical methods.